Surviving as a Software Developer in the AI Era
A software developer today faces multiple challenges and pressures in their daily work, including: keeping their job secure amid the rise of AI, learning new AI tools and integrating them into their workflow, staying up to date with every new tool that enters the market, and, amid all this, focusing on the essentials—developing software that is correct, efficient, and maintainable
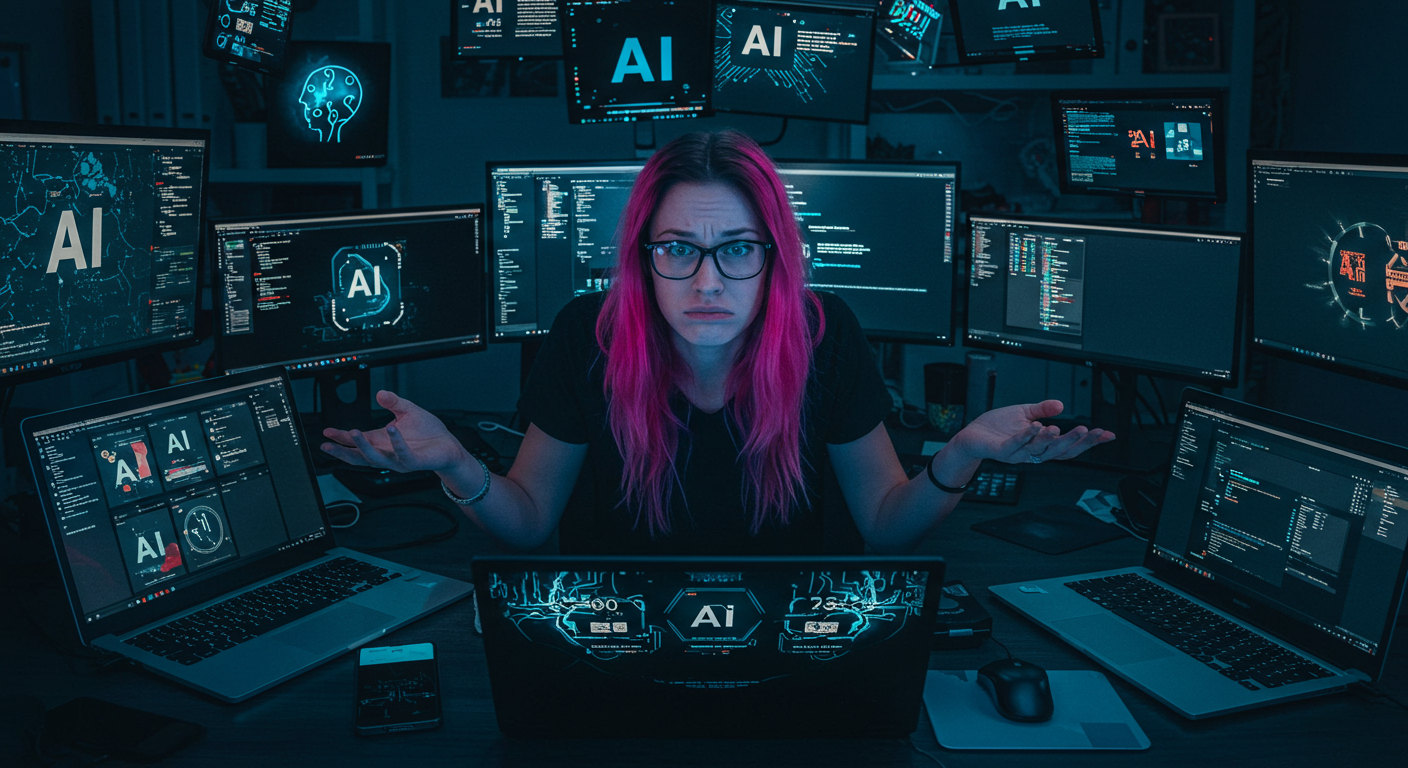
So, how does a contemporary software developer survive this storm of AI tools that haunt them and, in many cases, interfere with their work rather than collaborate? That’s what this article is about.
Relax
As a first recommendation: relax. Try not to stress about using everything the market has to offer (because there will always be a lot, and there will always be something new you’re not using). Instead, focus on continuing to do your job. You can integrate AI-based tools where it feels organic, natural, and useful, but forcing yourself to try every new tool that comes out and attempting to use it in every feature you develop is probably a recipe for disaster. Rushing to incorporate AI without taking the time to understand and reason through what you’re doing will likely be harmful to both your work and your career.
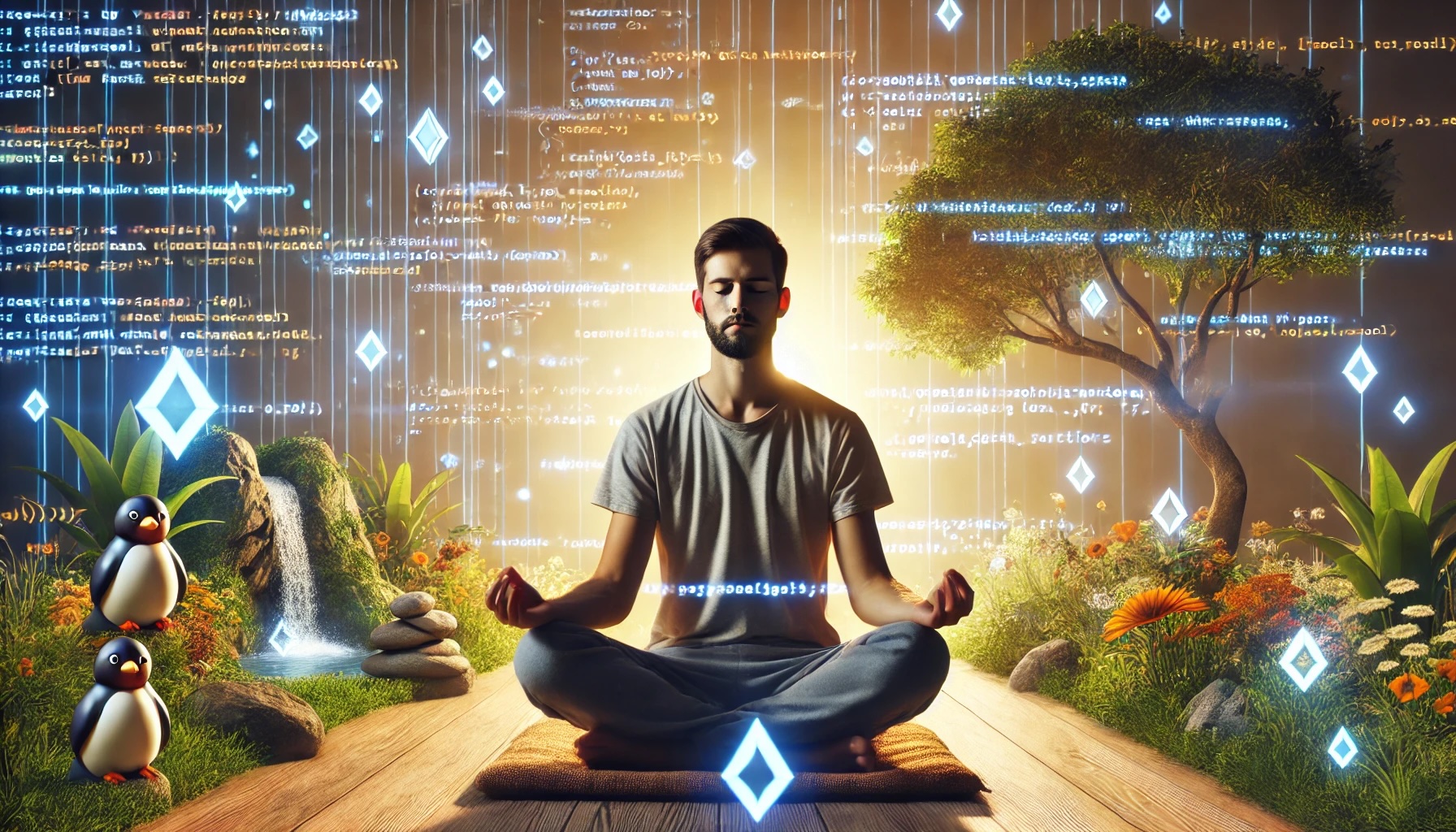
Credits: DALL·E 3 (https://openai.com/index/dall-e-3/)
In the short term, AI won’t take your job, but not incorporating AI tools could leave you without one in the medium term—just like failing to keep up with new languages, frameworks, and trends in software development (which has been happening for the past 40 years). Think of AI tools as a new technology that has emerged in the market—one you should adopt to stay up to date. The best thing you can do is learn to integrate AI tools organically to improve the work you’re doing today.
AI Tools for Developers
Now, if I’ve convinced you so far, you might be wondering: Which of the hundreds of AI tools for software development should I use? The answer, as in many cases, is: it depends. On what? Your seniority, the type of developer you are, your preferences, and several other factors. We’ll analyze some scenarios later.
But first, let’s take a quick look at the types of AI-assisted development tools available on the market today. Below we provide a non-exhaustive summary of the main categories of AI-powered developer assistance tools, along with some examples of each:
Chatbots
These are interactive chat models where you can converse with a text-generation model (LLM), like the well-known ChatGPT. While they aren’t specifically designed for developers, we include them because they are among the most widely used and are almost all optimized for code generation.
Examples: ChatGPT, Claude, Gemini, Grok, Perplexity, Microsoft Copilot, Deepseek
Autocompletion Assistants in IDEs
These are assistants integrated into popular IDEs, usually available as plugins, that suggest code while you type. Some, like GitHub Copilot, have evolved into embedded chat assistants that allow you to enter specific prompts, overlapping with the next category.
Examples: GitHub Copilot, Supermaven, Codeium Supercomplete
Code-Generating IDEs
These are fully-featured IDEs that come with built-in capabilities to generate code and even entire applications.
Examples: Cursor, Windsurf, Trae
Webs/SaaS Platforms
These tools function similarly to the previous category but are web-based instead of standalone applications. They allow users to generate complete applications using prompts and, in many cases, deploy them as well.
Examples: v0 (de Vercel), Lovable, Replit, Bolt
APIs
This category includes low-level APIs (usually REST) that provide programmatic access to LLMs.
Examples: OpenAI, Anthropic, Google/Gemini, Grok
Libraries for Building AI Agents
These are code libraries designed to help developers create autonomous agents that can perform tasks supported by AI models.
Examples: PydanticAI, LangChain, AutoGen, CrewAI, Agno
A special note goes to the category of “Full IDEs with Code Generation.” These IDEs are currently very popular (as of March 2025) because many people use them for the well-known “Vibe Coding.” This means describing the requirements of a software product in plain text, letting the IDE generate the entire code, testing it, tweaking the prompt if necessary, and continuing to iterate this way—without stopping to review, understand, or correct the generated code. Instead, developers trust the AI and keep “programming” based on what feels right. The term was coined by Andrej Karpathy, one of OpenAI’s founders, in this post on X.
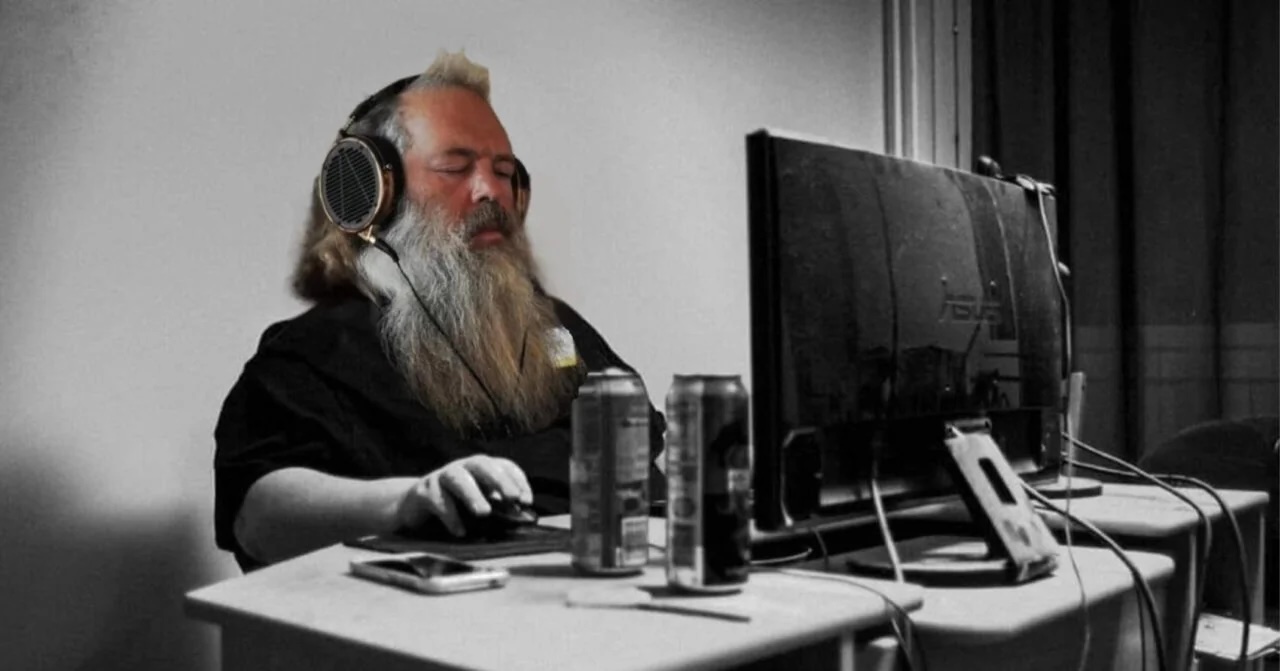
Credits: Reddit
So, What Should I Use?
If you’re a software developer, you’re probably wondering—after that mountain of tools we just summarized—what exactly should I be using?
As we mentioned earlier, the answer is highly personal. However, below are some general recommendations for AI tools based on your level of seniority. Of course, everything can be adjusted according to your preferences, needs, project type, technologies used, and other factors.
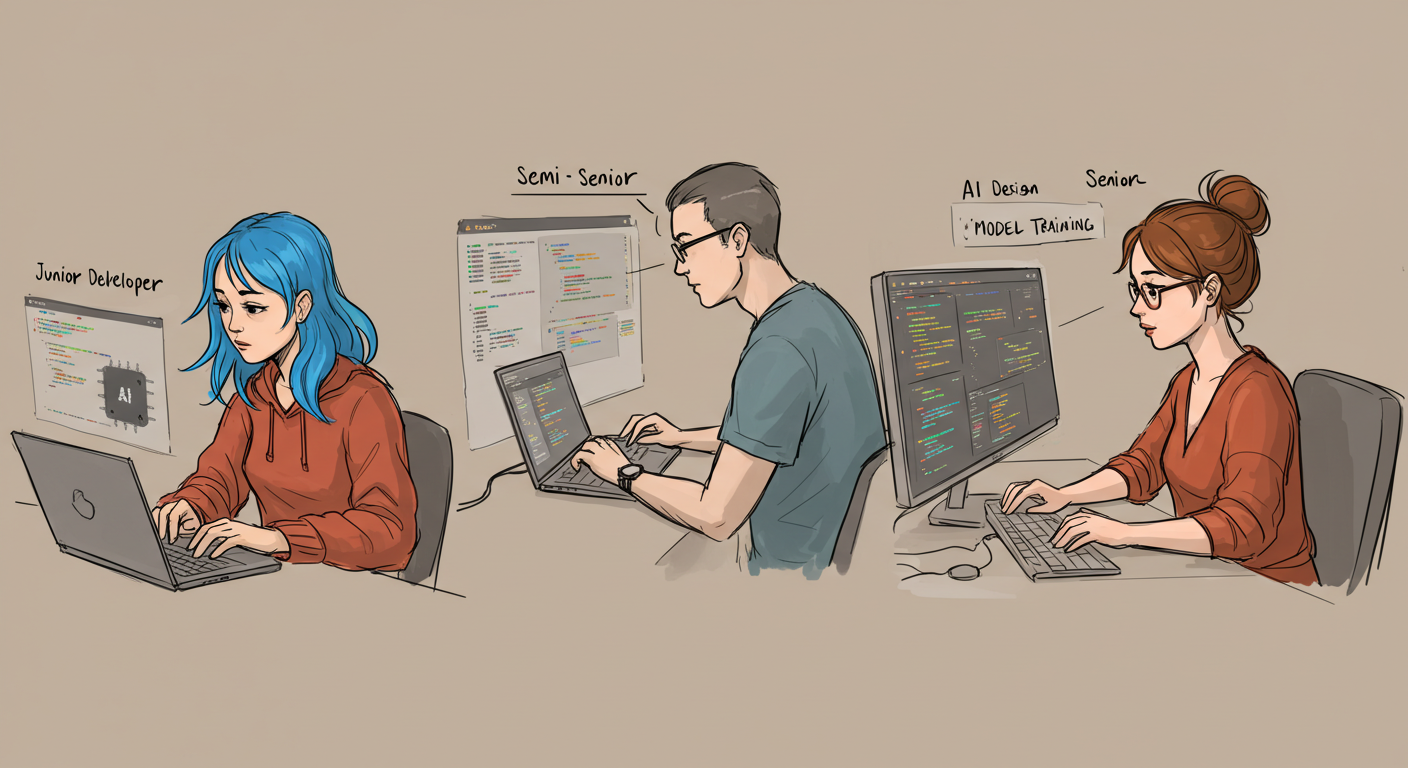
Credits: Google ImageFX (https://labs.google/fx/tools/image-fx)
What Should I Use If I’m a {seniority} Developer?
If {seniority} == “Junior/Trainee”
In this case, we recommend using AI tools with great caution and limitation. Keep in mind that you’re likely learning multiple things at the same time—a programming language, a framework, programming theory, and more. Overusing AI at this stage can hinder your learning process, but there’s good news: it can also empower and accelerate your journey to becoming a better developer.
At this point, the best approach is to primarily rely on tools from the “Chats” category and take advantage of their conversational capabilities to use them as mentors in your learning process. While they can’t fully replace a great human mentor, they can complement your learning. Make use of the chat’s memory to have extended conversations, digging into anything you don’t understand or find unclear. Instead of using prompts like: “Generate a React component for a to-do list.” you can use prompts that encourage learning, such as: “Please explain step by step how to write a React component for a to-do list. Walk me through the code line by line.” After reading the response in detail, you can ask follow-up questions about any parts that are unclear. This way, you can use AI as your personal programming tutor.
It’s not advisable to rely too much on other tools at this stage, but “Autocomplete Assistants in IDEs” can be useful for writing self-documented code. For example, with GitHub Copilot, in a new file, you could write the following comment:
/* Fetch all users from the database, sort them by last name in memory, then transform them into a JSON, commenting on each line of code*/
After this comment, GitHub Copilot will start generating the code. This is convenient because you don’t have to leave the IDE, and the comments can help you understand the generated code. However, chat-based AI tools tend to be more effective for these types of tasks since they allow for follow-up questions.
As you progress in your career, you can start incorporating other AI tools when you feel confident in your ability to understand the code that chat tools generate.
If {seniority} == “Semi Senior”
In this case, the previous recommendations still apply, but it’s also advisable to start enabling autocomplete tools in your preferred IDE. However, always make sure to read, understand, and validate the generated code instead of blindly accepting it. The goal is to leverage AI tools to speed up development while continuing to learn and acquire new knowledge.
It’s also a good idea to start using chat-based AI tools to generate small components, functions, or classes—things you could write from memory or by quickly referencing documentation, but that an LLM can generate even faster.
Again, never forget to read the code, understand it, test it, and document anything that isn’t obvious. LLMs make mistakes and sometimes produce nonsensical output, which can introduce bugs without you realizing it.
Another interesting way to use AI at this stage of your career is to ask it to review your code and provide recommendations before submitting a Pull/Merge Request. You can do this by copying and pasting your code into a chat tool or using AI-powered code review tools integrated into your IDE.
Similarly, you can ask these tools to write unit tests for your code. You could even describe a problem and ask the AI to generate only the test cases, so you can complete the code and validate it by ensuring the tests pass (AI-guided TDD). This can be a great way to challenge yourself and improve your mastery of the language or framework you’re working with.
If {seniority} == “Senior”
At this stage, we assume you have a solid grasp of the languages, libraries, and frameworks you work with, so you can freely use any of the AI tools we’ve discussed. In particular, AI-powered IDEs can significantly boost your productivity and help you become a “10x Engineer.” However, be mindful: it’s important to review the generated code, not just to ensure it meets your requirements but also to maintain a deep understanding of the codebase you’re building and to validate its robustness and quality. The less you understand the code you’re writing, the closer you get to “vibe coding”, which increases risks (we’ll discuss this more at the end of the article).
As we’ve mentioned, preferences are highly personal. For example, the author of this article prefers to write function/class/component headers first and let autocomplete tools generate the initial code, accepting what aligns with the intended logic and manually adjusting anything that doesn’t. This approach allows AI-assisted coding while still guiding the structure, form, and style of the code. However, in some cases, it’s more convenient to simply ask a chat-based AI to generate what you need—like writing a regular expression to validate an email—and copy-paste the result into your code.
As a general recommendation for any Senior developer, we suggest testing at least one tool from each category for a while to determine what works best for you and to what extent you prefer using them. The goal is to find the optimal set of tools that help you work as efficiently as possible while continuing to enjoy coding.
Risks
As we’ve mentioned throughout this article, overusing AI tools for development comes with some risks. Here are a few key concerns:
- Generating code with hidden bugs/errors that may not be immediately obvious
- Creating inefficient or highly coupled code, making future modifications difficult
- Producing code that is hard to maintain and debug, leading to long-term technical debt
- Introducing security vulnerabilities—or worse, being exposed to malicious code injection from LLM-generated outputs. This happens when the AI-generated code contains elements that could leak sensitive information from your application to third parties
- In large projects with significant amounts of AI-generated code, you may end up with inconsistent design patterns, as most AI models work with a very localized context (i.e., the files being edited) due to limitations in their context windows and cost-saving measures.
Regarding the risk of maintainability issues, one might think: I won’t need to maintain the code because AI can always do it for me—in other words, I don’t need to understand the code if AI understands it for me. This is true to some extent and could work if one is willing to rely heavily on AI. However, as a product grows, it becomes increasingly difficult to sustain and achieve good results. In such cases, it is recommended to document design, functional, and architectural decisions and provide these files to AI models. Typically, .MD files are used to write this documentation within the project, along with specific configuration files like .cursorrules in Cursor, which indicate where the documentation is located and define templates for standard files. This article explores these strategies in more depth. While this development approach is possible, it is still in its early stages, and the long-term maintainability of AI-generated code remains uncertain. It is also unclear whether LLMs’ memory windows can effectively handle large codebases—as the code volume grows, they tend to make more mistakes.
As a general recommendation to mitigate these risks, we advise using AI tools wisely—always reviewing and understanding the generated code. Treat them as a support for your work, not a replacement for it. Let’s show AI that we can use it to become better at what we do.
Outro
As a final note, we want to clarify that all opinions expressed in this article are the author’s personal views, and each reader is free to disagree, embrace vibe coding, and even wish to be replaced by AI in the future 😉.
Lastly, we’d like to share that at Teamcubation, we’ve been supporting junior and trainee developers on their learning journeys with personalized mentorship for over three years. Recently, we’ve added specialized programs to accelerate AI adoption in development teams. If you’re a developer or work with developers, we invite you to learn more about our services: https://teamcubation.com/.
Disclaimer: This article was entirely written by a human—word by word, letter by letter—without any AI assistance. Can you believe it? What a strange thing!

CTO at Teamcubation. Follow me on LinkedIn